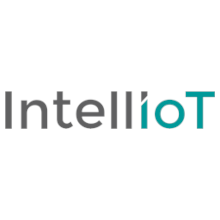
Finding a parking spot in urban areas is a pervasive issue worldwide, leading drivers to waste
valuable time and fuel each year. The search for parking contributes to increased traffic
congestion, as visitors circle around looking for a spot and also causes delays and
inconvenience for drivers running late for appointments or events. Moreover, the associated
energy consumption contributes to air pollution and greenhouse gas emissions, worsening the
issue of climate change.
The EU-funded project INTEL ANN aims to address these challenges by providing drivers with
predictive information on possible areas where they can find available parking spots. The
proposed solution leverages big data and machine learning algorithms to analyze information
from in-ground IoT sensors and predict parking availability in a city. By offering real-time
information on parking availability, the system aims to reduce the time, inconvenience, and
environmental impact of parking. This solution will be piloted in the city of Kalamaria in
Thessaloniki, Greece, where a hub of 150 in-ground parking occupancy sensors has been
installed to monitor parking traffic (IN/OUT per parking space).
To meet the above objectives, INTEL ANN will utilize one year's big data related to parking
occupancy. This data will be analyzed based on various parameters, including:
● Fixed time parameters
● Time-variable parameters
● Climate-based parameters
● Traffic-based data on the streets (e.g., traffic overloads)
● Bus stops related data
The system will further scale up to accommodate large metropolitan areas with a significant
number of parking spots. This will involve partitioning the metropolitan area into distinct parking
areas, each of which will be served by an edge-node responsible for collecting data from nearby
sensors. The system will utilize federated learning to analyze this data and provide accurate
predictive information through a mobile application to drivers in real time.